Monte Carlo Simulation
- RICHA RAMBHIA
- Nov 17, 2022
- 3 min read
Monte Carlo Simulation:
Monte Carlo simulation is a method which is used to model the probability of different outcomes in the event which cannot be easily predicted due to the intervention of the random variables. Monte Carlo simulation is also known as multiple probability simulation which can be used to tackle a range of problems. In a Monte Carlo simulation, the process involves assigning multiple values to an uncertain variable in order to achieve the multiple results and then averaging those results to obtain an estimate. Thus, in this method the simulation focuses on constantly repeating the random samples to achieve some sort of results.
The method of the Monte Carlo simulation is that it takes the variable that has uncertainty and then assigns it to a random value. The model is then run and the result for the same is provided. Now, this process goes on repeated over and over again while assigning the variable with many different values. After the simulation process is complete, the results of all are averaged to provide an estimate. This is how the Monte Carlo simulation works. Therefore, Monte Carlo simulation is a mathematical method for calculating the multiple possible outcomes which occur in an uncertain process through the repeated random sampling. (“Monte Carlo Simulation”).
Example of Application of Monte Carlo Simulation:
Let’s consider an example where the rTSR award’s vesting will be based on the subject company’s stock price relative to a group of four peer companies. The vesting percentage is based on rank of return over the measurement period as shown in the below figure. (“Monte Carlo Simulations: An Example of Application”).
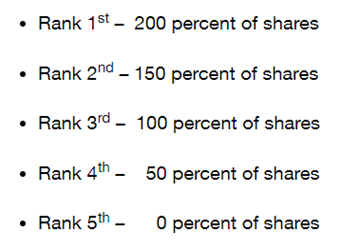
Now, to get the value of the rTSR award, it is required that we simulate the stock price of the subject company which can be done using the Monte Carlo Simulation. It consists of a large number of trials in which there is a new set of variables that are selected based on the defined distributions. Thus, in this example one trial would consist of a stock price path between the valuation date and maturity for the subject company which would represent one potential outcome or scenario in each case. And, to simulate each company’s stock price we have the following formula:
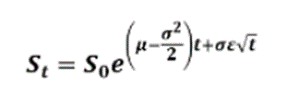
To calculate a Monte Carlo Simulation in excel one can model possible movements of asset prices by analyzing historical price data to determine the drift, standard deviation, variance, and average price movement as these are the building blocks of a Monte Carlo simulation.
Another example of a Monte Carlo simulation that can be considered is to create a work schedule for a research and development project. Here, the work schedule is created, and the duration of each activity is estimated and calculated as shown in the below table.
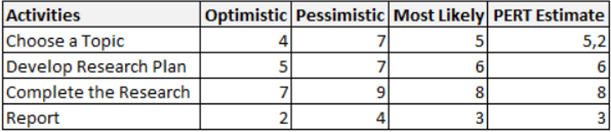
Now, after performing the Monte Carlo simulation the results we obtain are shown in the table below.
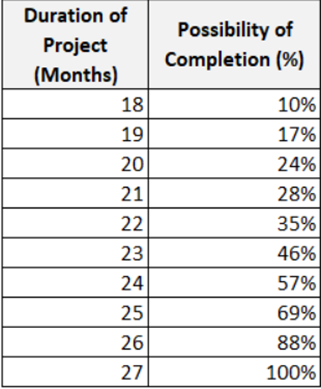
(Simosa 2018)
These results are analyzed further, and we observe that the probability of completion of project in the best case is the lowest and in the worst case is the highest. The simulation thus provides a number of results to improve the decision-making process.
Challenges in the Monte Carlo Simulation:
Monte Carlo simulation is very prominent in many fields where it provides complete solutions, but despite the successes it still has some flaws. The simulation is often unable to predict or assess the obvious dynamics within a system to equal success with each subset. The localized variables are overlooked because of a sense of inability to identify causality. And so, the underlying processes that occur within the simulation must closely model the processes that occur in reality. The simulation method is time consuming and a complicated method for quantitative risk analysis. While performing the Monte Carlo simulation there should be enough samples or the input sets to make sure and have a realistic result. Another challenge that one can face with respect to the simulation is that the correct analysis and results will depend on the quality of the estimates and thus that needs to be perfect. Monte Carlo simulation is a method which gives a number of results based on the probabilities and does not give the actual result. Therefore, the point here is to have enough sample input to conduct the simulation and to know that the result is based on the probability and not on the actual results.
References:
Kenton, W. (2021, October 4). Monte Carlo Simulation. Investopedia, from https://www.investopedia.com/terms/m/montecarlosimulation.asp
Monte Carlo Simulation. (n.d.). WallStreet Mojo, from https://www.wallstreetmojo.com/monte-carlo-simulation/
Monte Carlo Simulations: An Example of Application. (2018, April 9). A&M, from https://www.alvarezandmarsal.com/insights/monte-carlo-simulations-an-example-application
Simosa, F. (2018, February 14). Monte Carlo Simulation. Project Cubicle, from https://www.projectcubicle.com/monte-carlo-simulation-example/
Comments